Superfast AI 1/16/23
Game-playing AI, Anthropic's Claude, Google's Muse, and the future of AI with Apple, Amazon, Meta, Google and Microsoft.
2023 is already off with a bang! Today we’ll cover game-playing AI, Anthropic's LLM (Claude), Google’s image model (Muse), and the future of AI with Apple, Amazon, Meta, Google and Microsoft. Let’s dive in!
The Headlines
Want a 30-second run down on 2023 so far? Here are the headlines (link):
Microsoft bringing GPT to Bing, Word, Powerpoint, Outlook (link)
The Microsoft and OpenAI deal (link)
Google's text-to-image model Muse (link)
Stability AI teasing DeepFloyd / IF (link)
Apple's AI audiobooks (link)
Beta testing Anthropic’s new LLM, Claude (link)
OpenAI pilots a premium pro version of ChatGPT (link)
DeepMind's DreamerV3 mining Minecraft diamonds (link)
Microsoft VALL-E, which can clone your voice using just a 3-second clip (link)
DeepMind's Sparrow coming to private beta in 2023 (link)
Meta ReVISE, which can read your lips (link)
GPTZero, which detects a lot of AI-generated text (link)
ICML bans the use of generative AI in AI papers (link)
🗞 News
The future of AI in Big Tech
Ben Thompson wrote a deep dive on his blog, Stratechery (link). Here are the key takeaways:
Apple: Apple will continue to invest in open source capabilities with AI. Last year, Apple updated their iOS system to accommodate Stable Diffusion directly on Apple Silicon devices, which is preferable to web-based (online) applications for privacy and connectivity reasons (i.e. the ability to run off-line without wifi).
There is additional discussion about about how open source generative AI models (like Stable Diffusion) will beat out private models (like DALL-E) in the hardware/software flywheel.
Amazon: AWS will continue to benefit from compute/GPU needs for AI training. Amazon, however, still competes with Microsoft for GPU/cloud customers, and Microsoft has won over one of the biggest players in the space, OpenAI.
Meta: Meta should leverage AI to improve their ads business: understand which 1) users to target, 2) ads perform well, and 3) ads convert into purchases. As Meta dives deeper into hardware with their VR offering, they might also consider developing a server chip in-house.
Google: Google is rumored to have a conversational AI chat product and a image generation model that they have yet to launch publicly. It will be interesting to see how Google incorporates AI in its search offering moving forward.
Microsoft: Microsoft is well-placed as the exclusive cloud provider for OpenAI. The inclusion of ChatGPT in the Microsoft productivity suite (Word, Excel, PPT) and in Bing’s search engine bodes well for Microsoft. It will be interesting to see how Microsoft uses these opportunities to generate revenue from their usual subscription offerings.
LLM chat products
What’s the scoop on Anthropic’s LLM, Claude? (link)
Claude is Anthropic’s alternative to OpenAI’s ChatGPT
Claude rambles more than ChatGPT, except in response to trivia questions where it outperforms ChatGPT, and reduces additional “fluff” in the answers
Claude can tell better jokes (a must-try for a future Superfast AI newsletter!)
Claude is worse at programming and math than ChatGPT
Claude is a ‘constitutional AI’, which means its output is constrained by a set of rules (or a constitution) that aligns with human values. This includes maximizing positive impact, minimizing harmful advice, and respecting the user’s freedom of choice
These constraints makes sense given Anthropic’s focus on AI safety and alignment
Related: DeepMind plans a beta launch of Sparrow, their LLM chatbot, in 2023 (link)
Text-to-image generative models
What is Muse? (link)
Google’s Muse is an image generation model (like DALL-E, Stable Diffusion and Midjourney). The big improvement with Muse is that it relies on fewer computational resources and creates better image outputs than other image generators
Muse uses parallel decoding in token space, which differs from diffusion models and autoregressive models. This innovation allows Muse to perform more efficiently than other image models without losing visual quality
Diffusion models use progressive denoising (think about removing static)
Autoregressive models use serial decoding (step-by-step, rather than in parallel)
Muse improves over other image models via:
High-performance: Muse matches or outperforms other state-of-the-art models on CLIP and FID evaluations
Speed: due to the parallel sampling method, Muse performs faster than Stable Diffusion and Imagen (Google’s other text-to-image model)
Cardinality: improves on the number of objects in an output
Compositionality: improves on the location of objects in an output
Text rendering: improves on forming text in images (which image models typically struggle with)
Check out a fuller dive here:

3 Headlines and a Lie
The headlines in AI keep getting crazier. Can you guess which of these headlines is fake? (Answer at the bottom of the newsletter).
GPT-4 beta generated a catfishing strategy used to steal this man’s wife over WeChat (link)
ChatGPT conducts research and is co-authored on a paper (link)
AI named CEO of a 3,000 person, $10B company (link)
ChatGPT is Reid Hoffman’s most recent podcast guest (link)
📚 Concepts & Learning
Games, games, games
DeepMind announces DreamerV3 (link)

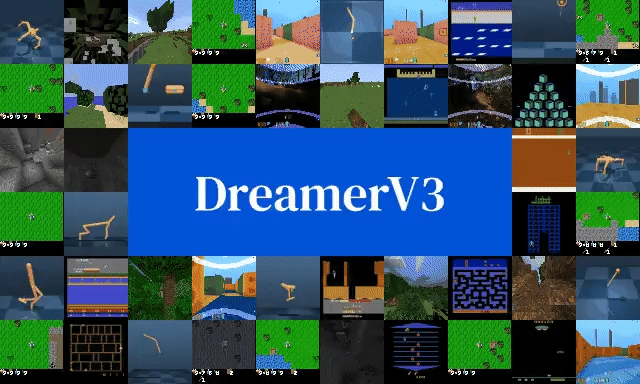
DreamersV3 is the first algorithm to collect diamonds in Minecraft from scratch without human data or curricula, a long-standing challenge in artificial intelligence
This is an improvement on previous training methods that required human feedback, or specific curriculum training to guide the model in the right direction.
What is curriculum training? We’ll do a deeper dive on curriculum training in the coming weeks. For now, think of training curriculum like a school syllabus. It makes sense to learn arithmetic before calculus. The same applies to model training. If you change the order that you introduce concepts to the model, it can learn better/more efficiently.
Related: In the book The Alignment Problem by Brian Christian, there is a section on encouraging models to do (random) exploration in the game Montezuma's Revenge to move towards (and collect) sparse game rewards. These training methods are important to AI progress, because many tasks/goals we have involve sparse rewards: when you cook, you’re rewarded for delivering the final dish, not chopping the onions. In Montezuma’s Revenge, you’re rewarded when you find the cave of treasures, not for running down one path over another. Here’s a quick YouTube video you can watch on the topic (link).
Also related to AI progress in gaming: Last year, Meta AI researchers developed 'Diplodocus’, a model that can beat expert human players at the coordination/negotiation game Diplomacy. Even more impressive, human players preferred coordinating with AI over other human players. Read more here.
One more: Researchers at Meta AI took their Diplomacy-game research further by building Cicero, a model that coordinates with humans using language model capabilities. “CICERO achieved more than double the average score of the human players and ranked in the top 10 percent of participants who played more than one game.” Read more here.
This reminds me of this map in Max Tegmark’s book Life 3.0. Tegmark describes AI progress looking like the rising water levels over a varied topological landscape. At the time of publishing, the items “underwater” were subjects that AI had already reached human-level intelligence on, like successfully competing with expert humans in the game of Go. We also saw this with IBM Watson’s success in the game of Jeopardy. The “higher ground” items such as driving, management and programming would successively be achieved based on their elevation on the map.
I wonder how Tegmark might update this map with new information about AI capabilities today! We still haven’t fully deployed self-driving cars (a lower elevation item), while programming and book writing seem increasingly achievable in the near future based on the recent advances of LLMs! Food for thought.
🎁 Miscellaneous
Wolfram|Alpha
Wolfram|Alpha teaches math to ChatGPT (link). Highlights:
ChatGPT fails to correctly rank order a list of countries by population
ChatGPT contradicts itself mid-paragraph
ChatGPT hallucinates facts about the world
ChatGPT fails to understand a metaphorical query: How many calories are there in a cubic light-year of ice cream?
Food for thought
A thread by Tyler Cowen and Nathan Labenz on AI (link). Key takeaways:
Pruning for sparsity – turns out some LLMs work just as well if you set 60% of the weights to zero (though this likely isn’t true if you’re using Chinchilla-optimal training)
Distillation – a technique by which larger, more capable models can be used to train smaller models to similar performance within certain domains – Replit has a great writeup on how they created their first release codegen model in just a few weeks this way!
Distributed training techniques, including approaches that work on consumer devices, and “reincarnation” techniques that allow you to re-use compute rather than constantly re-training from scratch
Deepfake yourself. It’s worth it
DoNotPay, a self-described robo-lawyer company that assists with customer service and litigation issues for its clients, soon plans to offer generated voice options that lets users clone their own voices. Use this on your next customer service call with your airline if you’re on the receiving end of a flight cancellation (too soon? Holiday flight season was rough).
Read the report with Vice here.
Related: companies that are working on voice cloning include Resemble AI, Eleven Labs, Apple and Microsoft via VALL-E.
Just for fun
Celebrating Lunar New Year next week? You won’t want to miss this AI generated LNY-themed video by Karen X Cheng using NeRF + Fields Editor:
Ryan Reynolds makes and ad with ChatGPT:
Six OpenAI rivals Google and Microsoft are watching (link)
That’s it! Have a great week and see you next Sunday! 👋
3 Headlines and a Lie:
The catfishing strategy headline was the lie! The rest are, in fact, true.